Computer vision meets protest research
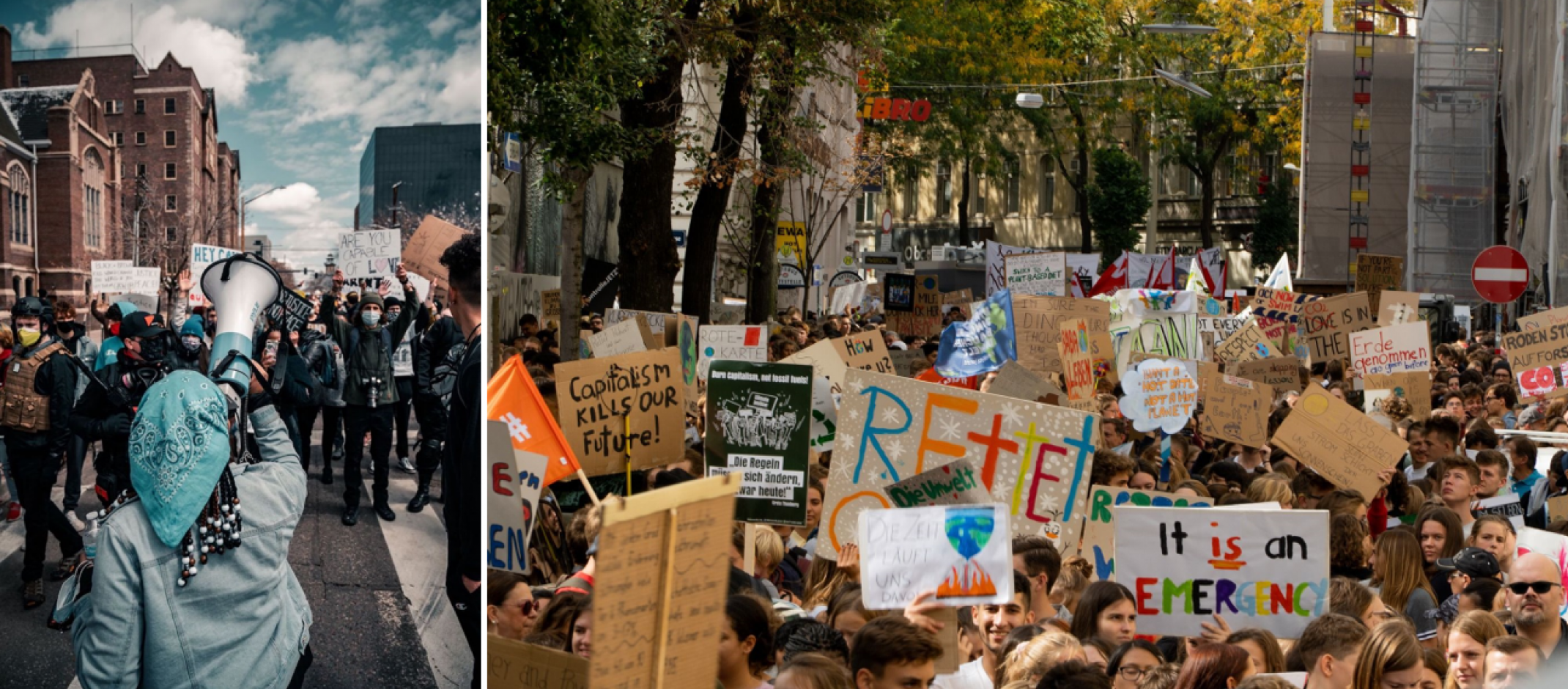
Stefan Scholz bends over his laptop and studies the screen, which shows a confusing sequence of letters, numbers and brackets – a Python script used by software developers, for example. Scholz scrolls to a line and types a short command. The effect is enormous: The photo projected onto the screen changes instantly from a normal image of a crowd of people to a brightly coloured hodgepodge of key terms, rectangles and numbers.
What Stefan Scholz, a doctoral researcher at the University of Konstanz, is doing is called social science image analysis. Computer-aided methods that can be used to analyze and categorize images have existed for some time now. At the Centre for Image Analysis in the Social Sciences (CIASS), however, researchers are now blazing a new trail. Using an established method from the field of computer vision (see blue info box), they aim to advance the analysis of images in the social sciences.
Computer vision
Computer vision is a field of artificial intelligence (AI). The goal is to train computers to recognize what is being represented in visual media such as images or videos. There are different methods for obtaining meaningful information. At the CIASS, researchers use one of these methods, a segmentation method, which they adapt for image analysis in the social sciences.
The interdisciplinary research team, consisting of experts in computer science and political science, is currently analyzing protest images from social media. Hundreds of thousands of images have already been viewed and categorized by hand. This is standard procedure for machine learning: The machine needs to be trained by humans. That much is clear. But why is a new method required now? Nils Weidmann, who initiated the foundation of the centre, explains:
![]()
"The computer vision methods used so far can predict quite well whether a photo depicts a protest. However, we do not know which objects the computer uses to classify a photo as a protest image. So transparency is the problem we want to solve".
Nils Weidmann
In computer vision, the main aim is to increase the recognition rate of the programme, and it is of secondary interest why artificial intelligence (AI) recognizes a coffee cup as a coffee cup. In the social sciences, however, this is precisely what is problematic. Social scientists use classification algorithms as a data basis and want to understand: Could it be, for example, that the AI is using the wrong objects and thus distorting further scientific analysis? Or do we maybe learn more about the image when we see it "through the eyes of the computer"?
Which brings us back to the colourful hodgepodge from the beginning: Stefan Scholz's command did not apply a lollipop filter to the crowd, but produced the result of a protest analysis in a matter of seconds. Unlike conventional methods, which only colour certain areas, such as protest posters, red (think of thermal imaging cameras), various objects on the image are now also recognized. Thanks to prior manual coding, the AI now "knows" which combination of objects characterize a protest image.
This is what is so innovative, because objects can be counted. As a result, the researchers get an abstract representation of an image that only describes which and how many objects are in an image. "In this way, we translate the images into a generally understandable language of objects, such as 'the image shows two protest posters and three security forces. We use these, in turn, to predict whether it is a protest image", explains Nils Weidmann. Images with a certain combination of these objects, for example, are very likely to indicate a protest. Weidmann summarizes the segmentation process: "Ultimately, we standardize the process to a vocabulary of entities and understand why artificial intelligence predicted ‘protest’."
Methodological background
The protest images were selected from existing protest data sets. These data sets provide information on which countries and time periods have seen protest activity. Overall, the researchers analyzed photos from the X platform (previously known as Twitter) from 14 different countries for the project "Transparent Classification for Protest Coding from Images" and manually coded 190,000 images of protests.
Picture gallery: examples of how different computer-aided methods are used to analyze images
Heat map method:
Comparable to a thermal image, certain areas that indicate protest are marked (in red). In the image on the right, this method recognizes the protest signs in the foreground, but otherwise mainly the surrounding buildings. This is where the transparency problem becomes clear: Why are these images classified as protest images?
Another method based on the heatmap principle:
In this case, the computer-assisted method does not detect a protest and the images would not have been included in the data collection.
***
The segmentation method:
This example shows the method used in the CIASS. The AI is provided with relatively few segment categories. The procedure recognizes these categories, but the result is imprecise without further categories. Using all of them together leads to the successful classification of a protest image. If (as in the example on the right) only people are detected, it could also be a picture from an outdoor shopping mall on a Saturday afternoon.
The method of the CIASS:
The computer has numerous additional categories available (such as banner, poster or signboard) and identifies them on the image. Combined they make it possible to conclude, with a high degree of probability, that the image shows a protest.
***
The comparison in the same image: Original image and computer analysis.
Some may wonder why all this effort is necessary. After all, the human eye very reliably recognizes protests or demonstrations. However, the millions of images that are published daily just on social media require machine support. "When analyzing images, we also face the challenge that protests can look very different in different countries", adds Stefan Scholz. Moreover, captions often do not clearly indicate that it is a protest image.
The advantages are clear: In addition to the sheer number of images that the algorithm analyzes in a fraction of a second, the computer-aided process classifies protests independently of text and language. And that is not all: Images as a data basis can often reveal subtle information that traditional sources in the social sciences (surveys, texts, etc.) do not catch. The material collected via crowdsourcing in social media can be used, for example, to track the emergence and escalation of a protest. Conventional media coverage usually only begins when a protest is already in full swing.
Eda Keremoglu sees enormous potential for the future of the method: "As soon as we go public with our initial research results, many social scientists will realize just how many possibilities image analysis offers. We anticipate completely new application possibilities".
More information on CIASS projects and image analysis in the social sciences is available on the CIASS website.
Information about the scientists
Professor Bastian Goldlücke
Bastian Goldlücke is a professor of computer science and head of the "Computer Vision and Image Analysis" research team. He develops new methods for gathering information from photos and video recordings.
Dr Eda Keremoglu
Eda Keremoglu is a postdoc in the research group "Communication, Networks and Contention" in the Department of Politics and Public Administration (University of Konstanz) and principal investigator in the Cluster of Excellence "The Politics of Inequality". Her research focuses on authoritarian regimes, information and communication technology, protest and repression.
Stefan Scholz
Stefan Scholz is coordinator of the Centre for Image Analysis in the Social Sciences (CIASS). While working at the centre, he is completing his doctorate with a focus on machine learning, image analysis and the analysis of social and political events. He holds a master's degree in "Social and Economic Data Science".
Professor Nils Weidmann
Nils Weidmann is a professor of political science, principal investigator in the Cluster of Excellence "The Politics of Inequality" and head of the research group "Communication, Networks and Contention". Weidmann has a background in social science and computer science.