A new era in the behavioural sciences
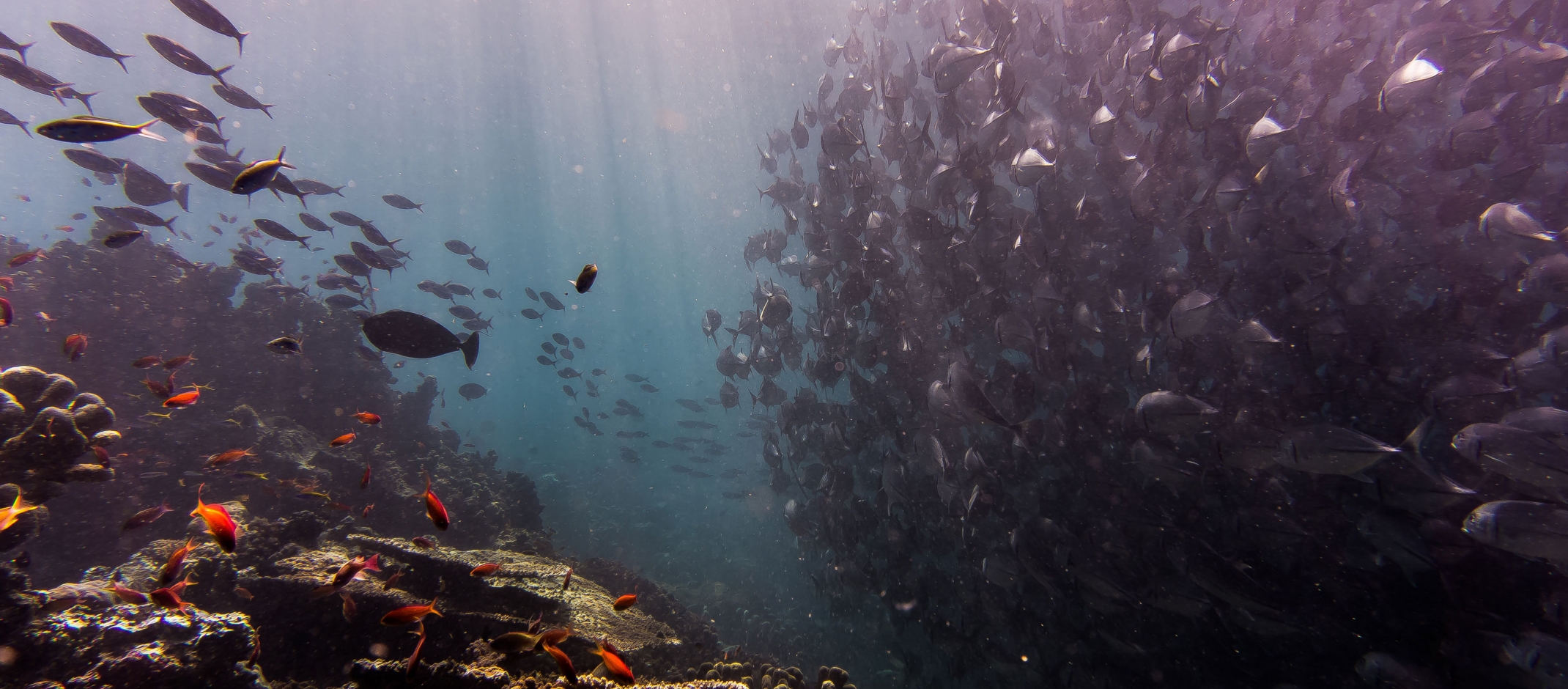
Iain Couzin can’t remember the number of ants that he had painstakingly observed before he came to the realisation that he needed the help of a machine. It was the mid-90s, during his PhD, and Couzin was trying to study how ants organize themselves. With colonies comprising anywhere from 300-400 ants, he was vastly outnumbered. “The best I could do was to record the ants, then watch the video 400 times over focusing on a different single individual each time,” he remembers.
But Couzin wasn’t interested in single individuals. Couzin studies collective behaviour—the phenomenon that turns bird into flocks, fish into schools, and locusts into devouring swarms. In order to truly understand the mechanics of collectives, he needed a vastly improved sensory system with the capacity to zoom out from the individual to compute the entire swarm. It was at this point that he came upon the idea of augmenting his senses with artificial ones.
Today he is professor of biodiversity and collective behaviour at the University of Konstanz, one of the speakers for the university’s Cluster of Excellence “Centre for the Advanced Study of Collective Behaviour” and director at the 50-person Max Planck Department of Collective Behaviour that was the incubator for the independent Max Planck Institute of Animal Behavior founded in May 2019. His research team is at the leading edge of a machine-learning wave that is sweeping the behavioural sciences. By exploiting advances in fields of artificial intelligence — particularly deep learning and artificial neural networks — their work is revolutionizing the study of collectives by teaching computers to see what humans cannot: patterns amid the mind boggling complexity of animal collective behaviour.
Computer program for tracking animal movements in the lab
But back to the mid-90s and those ants. Because it was impossible to capture data from all ants simultaneously by traditional observation, Couzin turned to computer vision after realising that a computer could localise individuals for him far better and faster than any human ever could. So he wrote a computer program that would track the position and orientations of all ants within a colony. The program worked well enough that it was used to track other animals in the laboratory, like locusts and fish, over the next two decades. There were, however, obvious limitations to this approach. The most salient is that the program only really worked in situations where rules were very clear (darker pixels = animal; lighter pixels = background): for example, in the laboratory. Animals, however, usually occupy worlds where this approach falls apart. Backgrounds are never uniformly white, but cluttered with vegetation; individuals are rarely isolated, but huddled in fluid groups.
“For a long time, nothing much changed in terms of the data we could capture,” says Couzin. “Computers got faster, camera resolution improved, but the next real technological breakthrough didn’t arrive until very recently with the advent of deep learning.”
Deep learning, a field of artificial intelligence, is a method of teaching computational models using real-world data. Loosely inspired by neurons in the brain, it uses artificial neurons to carry out basic logical functions and to learn the way humans do: through life experience (i.e. from data) rather than pre-programmed rules (i.e. traditional algorithms). Just as the neurons of a toddler become stronger or weaker with reinforcement, an artificial neural network can also, through trial and error, strengthen relationships between neurons that lead to a correct result. The more layers of neurons—i.e. the “deeper” the network—the more powerfully the machine can accurately predict patterns in huge reams of data.
Take Couzin’s original problem of tracking groups of animals in the lab. While two overlapping fish in a tank would once throw off the tracking program, requiring a human to re-connect individual tracks using guess work, deep learning has virtually eliminated these errors. PhD student Tristan Walter has implemented software that can identify individual fish based on subtle differences in colour patterns on their backs that are invisible to human observers. “The technique can solve problems that humans cannot, like individuals hiding under a cover together for long periods, individuals going in or out of view, or just simply if individuals overlap,” says Walter. “We simply can’t tell the difference between fish A and B, but computers can.”
Collecting data on wild animals without using tags or GPS collars
But for the science of collective behaviour, the holy grail has always been to study animal groups in the wild. The powers of deep artificial networks—such as their ability to become highly specialised at tasks well beyond the capabilities of humans—means that detailed data can now be acquired from wild animals without even needing to attach tags or GPS collars. For the first time, the behaviours of large numbers of free-ranging individuals can be recorded simultaneously, objectively, and at high temporal resolution. In addition to vastly improving how much data is acquired, deep learning tools can in turn be used to analyse these highly complex data sets. A new era for research into animal behaviour is dawning – in the University of Konstanz’s Cluster of Excellence “Centre for the Advanced Study of Collective Behaviour”: where actions are quantified, subjective biases are removed, and the hidden is finally revealed.
In Kenya, Konstanz post-doctoral researcher Blair Costelloe is employing a variety of deep learning techniques to examine collective detection and information transfer in wild Grévy’s zebras. “The processes we’re studying were described decades ago and yet there are very few studies of them in natural animal groups simply because we haven’t previously had the ability to collect the necessary data,” says Costelloe.
Program can recognize zebras anywhere
The first step in collecting the data is to find the zebras. Costelloe captures video footage of natural herds using a drone that she flies 80-metres above the grassy plains of the Mpala Conservancy. Then, to identify animals in the video, PhD student Ben Koger employs a pre-trained convolutional neural network, developed originally by Microsoft programmers, that he fine-tunes with labelled images so it learns to recognize zebras almost anywhere—even partially concealed under trees. “If you were to use the traditional tracking method based on pre-programmed rules, you could never code every combination of rules for all possible scenes in nature,” says Koger. “Deep learning has the advantage that models have millions of parameters, which means that they can recognize a zebra in any scene provided they have been exposed to enough training images.”
Once the animal is detected in the scene, the next step is to record what it is doing. In other words, you need information on its body posture, as only then can tell you if the fish is escaping, the bird is preening, or the zebra is startling. For this, Costelloe has teamed up with PhD student Jake Graving who has developed state-of-the-art deep learning methods for estimating body posture of animals in the laboratory or in the wild. Graving uses deep learning techniques to help understand how different sensory stimuli mediate behavioural contagion to cause coordinated marching in the desert locust. His method involves training a network to identify locations of an animal’s body parts directly from images. For zebras, these joint locations add up to 9 keypoints (or “dimensions”); and for desert locusts it reaches 35. Naturally, this results in extremely complex data sets—what scientists refer to as high-dimensional data—so Graving’s next step is to apply more machine learning algorithms, known as dimensionality reduction and clustering, to compress these data into smaller sets that captures a more basic, interpretable description of what the animal is doing over time.
The aim is to create even more powerful methods for tracking animals
If this sounds complicated, it’s only the beginning. Hemal Naik is aiming to develop even more advanced pose estimation methods. In the “Imaging Barn” facility located in the MPIO Radolfzell, Naik is using deep learning to train a network to predict 3D body postures in birds directly from 2D video recordings—the first tool to provide pose information in 3D for non-human animals using these methods.
Finally, with basic descriptions of behaviour acquired, the scene is set for answering questions in animal behaviour that have previously been out of reach. Dr Alex Jordan, principal investigator in the Max Planck Department of Collective Behaviour in Konstanz and research group leader in Iain Couzin’s team, is tapping the potential of machine learning in order to shed light on the actual causes of behaviour. “Natural selection, the great tinkerer, is a comparative process, endlessly comparing one variation against another,” says Jordan. “But for humans, it is almost impossible to see the small scale differences in behaviour that might provide the raw material on which selection can act”. But machines and neural networks can see what scientists cannot. Jordan’s team uses supervised and unsupervised machine learning approaches to generate massive comparative datasets on all behaviours shown by species of fish in “Darwin’s Dreamponds” – lakes in Africa that boast an explosive radiation of fish body shapes, colours, and importantly, behaviours.
Machine learning delivers a far more objective and quantifiable tool for understanding collective behaviour in nature—a messy, impossible-to-control place that’s far from the scientific comfort of sterile petri dishes. “This is where machine learning outstrips any other approach, bringing natural behaviour to the age of big data,” he says. “Where before we struggled to collect adequate data to understand behaviour, now we face a different and far more interesting problem – what does it all mean?”